Anjaly Parayil
​
​
Motivated by decentralized approaches to machine learning, we propose a collaborative Bayesian learning algorithm taking the form of decentralized Langevin dynamics in a non-convex setting. Our analysis show that the initial KL-divergence between the Markov Chain and the target posterior distribution is exponentially decreasing while the error contributions to the overall KL-divergence from the
additive noise is decreasing in polynomial time. We further show that
the polynomial-term experiences speed-up with number of agents
and provide sufficient conditions on the time-varying step-sizes to guarantee convergence to the desired distribution. The performance of the proposed algorithm is evaluated on a wide variety of machine learning tasks. The empirical results show that the performance of individual agents with locally available data is on par with the centralized setting with considerable improvement in the convergence rate.
​
​
​
Observability Enhancement in Systems with Partial Observability:
​
This work addresses the problem of collecting information about system states
when the system itself is partially observable. Major research questions addre-
ssed in this work include:
1. What are the best set of measurements that give maximum information
about the states.
2. How to collect the measurements that maximize information content?
The system considered in this work is a set of aerial vehicles with measurements
being the angular separation between the vehicles. In this setting, the separation
between the vehicles (R) is normally unobservable. Major tools used in this
research are information-theoretic notions such as observability gramian,
An Illustration of a set of aerial vehicles
Fisher Information Matrix, etc. A key finding from this work is that all the measurements differ in the information content about the estimation process, and the information-theoretic notion represents a better way to select measurements to bring additional information. As seen in the Figure, separation R between the two aerial vehicles is crucial in this setting when the only information available is the angle between the vehicles.
​
​
​
​
More on Other Research, Coming Soon....
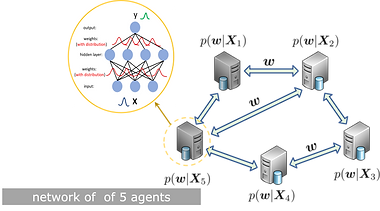
